Share This Article:
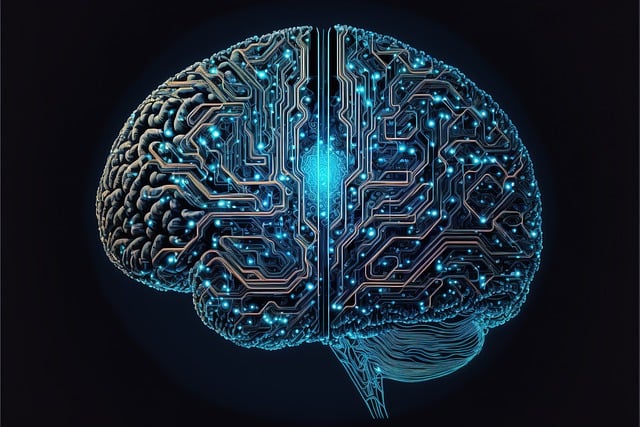
Sarasota, FL (WorkersCompensation.com) – Value based care and alternative payment models have been quite the experiment for healthcare the last few years. Used primarily by commercial, federal and state payers, payments using diagnosis-based risk adjustments accounted for more than $850 billion of healthcare payments in 2021. According a recent study published earlier this month in JAMA Network Open, new algorithms could be the key to more accurate calculations of risk adjusted payments.
Hierarchical condition categories (HCCs), created in 2004 by CMS, are used to assign risk scores that are utilized in calculating payments. Critics of HCCs state that the categories have changed little since 2015 when the implementation of ICD-10 began, bringing about more meticulous diagnosis information. The critics state that the HCCs don’t account for the large data sets now available due to technology advancements. Additionally, the vulnerability of up-coding, and fraud have been arguments used by those calling for an overhaul of HCCs and risk adjustment calculations.
Researchers from the University of Kentucky argue that current HCCs models that predict healthcare spending and other outcomes are used by the majority of payers to manage and issue provider payments despite well documented flaws and inconsistencies in the methodology. To address these concerns, the researchers tested whether a machine learning algorithm could be used to create risk adjustment models that take into account clinical logic while addressing potential up-coding incentives and predicting cost of care better than the current federal HCC models. Using Diagnostic Item (DXI) categories and Diagnostic Cost Group (DCG) methods, clinical and claims data for commercial enrollees aged 64 and younger from the Merative MarketScan Commercial Claims and Encounters Database was reviewed and compared to HCC models from the US Department of Health and Human Services (HHS).
DXIs were grouped into disease hierarchies with an assigned Appropriateness to Include (ATI) score to identify vague conditions and up-coding opportunities. The ATI scores were then used as predictors where the DXI was clinically mapped to the most serious level of disease, even in rare disease cases.
The researchers contend that considering current value based care models, new methods that can utilize and model over 70,000 diagnoses without creating invalid opportunities for up-coding is greatly needed. Usual models that estimate risk adjustment draw from repeated sampling of less than 1 million data sets, and classify with pre-specified sets of predictors. The researchers argue that such models are not accurate in predicting costs associated with rare conditions that may be outliers.
While the methodology eliminated vague diagnosis classifications and up-coding incentive opportunities, researchers found that the model dramatically reduced HCC underpayments for rare conditions when compared to HHS models. Additionally, the researchers found that while predictive models work well in healthy populations, or in cases where there are only a few diseases, the ability for standard risk models to accurately predict costs decreases as there are more morbidity factors. In these cases, the researchers believe that traditional risk models cannot calculate the cumulative effect of multiple diseases in a single patient. While the test algorithm was similar to the HHS model, the researchers found the test model was better at differentiating rare, higher-cost conditions and had greater overall predictive power.
arizona california case management case management focus claims cms compensability compliance conferences courts covid do you know the rule exclusive remedy florida FMLA glossary check health care Healthcare iowa leadership medical medicare minnesota NCCI new jersey new york ohio opioids osha pennsylvania Safety state info tennessee texas violence virginia WDYT west virginia what do you think women's history month workers' comp 101 workers' recovery workers' compensation contact information Workplace Safety Workplace Violence
Read Also
About The Author
About The Author
-
F.J. Thomas
F.J. Thomas has worked in healthcare business for more than fifteen years in Tennessee. Her experience as a contract appeals analyst has given her an intimate grasp of the inner workings of both the provider and insurance world. Knowing first hand that the industry is constantly changing, she strives to find resources and information you can use.
More by This Author
Read More
- May 03, 2024
- Chris Parker
- May 02, 2024
- NCCI
- May 02, 2024
- Frank Ferreri
- May 01, 2024
- Frank Ferreri
- May 01, 2024
- F.J. Thomas
- Apr 30, 2024
- Anne Llewellyn